AI-Powered Predictive Maintenance to Cut Downtime & Costs
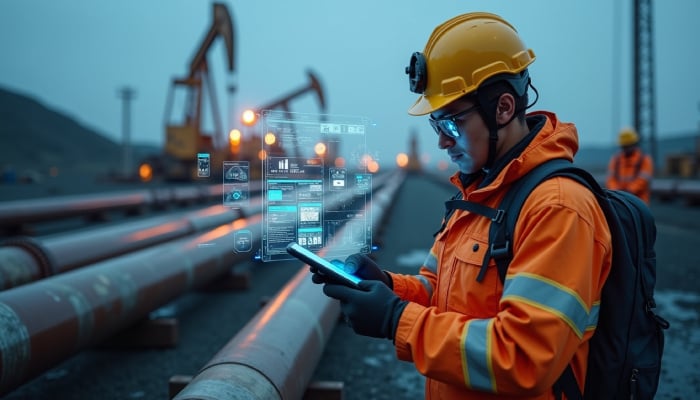
In industrial operations, every minute of unplanned downtime can translate to thousands—or even millions—of dollars in lost productivity, wasted resources, and delayed outputs. For industrial manufacturers alone, these disruptions amount to an estimated $50 billion in losses every year, according to a report by Deloitte, stemming from halted production, idle labor, missed orders, and a cascade of operational setbacks when critical equipment fails.
Predictive maintenance, supercharged by Artificial Intelligence (AI), has emerged as a game-changing strategy to combat these challenges. But beyond the broad promises, how is AI truly reshaping maintenance—and what does that transformation look like in complex, asset-intensive industries like chemicals, oil and gas, and manufacturing?
Why Reactive and Preventive Maintenance Fall Short
Traditionally, maintenance strategies have leaned on either reactive or preventive approaches. Reactive maintenance—fixing things only after they break—is costly, disruptive, and often leads to extended downtime. Preventive maintenance—scheduled servicing at fixed intervals regardless of actual equipment condition—helps reduce failures but frequently results in unnecessary part replacements, over-servicing, and wasted labor.
Predictive maintenance (PdM), in contrast, leverages real-time data from sensors and operational systems to anticipate equipment failures before they occur. This condition-based approach not only enhances reliability but also drives significant cost efficiency. In fact, organizations that have deployed predictive maintenance programs have reported up to a 40% reduction in overall maintenance costs, simply by addressing issues before they escalate into critical failures. The transition from time-based to data-driven maintenance is where AI unlocks exponential value—minimizing both risk and resource waste across industrial operations.
How AI Transforms Predictive Maintenance
AI doesn't just automate existing processes—it redefines them. Here's how:
- AI-Driven Condition Monitoring
AI algorithms continuously monitor equipment health by analyzing data from IoT sensors—temperature, pressure, vibration, etc. Unlike threshold-based alerts, AI models can detect micro-anomalies that indicate early signs of wear and tear. This leads to significantly earlier interventions. - Root Cause Analysis and Failure Pattern Recognition
Machine learning models identify recurring failure patterns and provide insights into why a component is failing—whether it’s due to operational misuse, environmental factors, or manufacturing defects. For industrial teams, this moves maintenance from being reactive to strategic. - Dynamic Maintenance Scheduling
AI systems can prioritize work orders based on risk, asset criticality, and operational impact. This ensures resources are allocated efficiently without disrupting core production lines. - Prescriptive Insights and Workflow Automation
Advanced AI doesn’t stop at prediction—it prescribes next-best actions. For example, if a critical asset is predicted to fail within 30 days, the AI system can automatically generate a work order, assign a technician, and check part availability.
This tightly integrated, intelligent loop of prediction, diagnosis, and execution is exactly the kind of digital transformation being driven by platforms like AI-first Connected Worker Platform.
Realizing Predictive Maintenance with Innovapptive’s AI-Enabled Capabilities
While AI's theoretical benefits are well known, execution is where most organizations falter. Innovapptive bridges this gap by combining mobile-first user experiences with AI-powered back-end capabilities. Here's how:
- Mobile Maintenance Apps for SAP and IBM Maximo: Maintenance teams receive real-time alerts and work orders on mobile devices, allowing instant responses to AI-driven insights. Instead of waiting for weekly checklists, teams act when failure risk spikes—right from the plant floor.
- Work Instructions with Embedded Predictive Alerts: When paired with sensor data, digital work instructions are no longer static documents—they evolve in real time based on asset behavior, guiding technicians with precision.
- AI Root Cause Analysis Engine: Innovapptive uses historical failure data and machine learning to generate root-cause insights. This significantly reduces troubleshooting time and prevents repeat failures across similar asset types.
- Maintenance Insights Dashboard: The platform aggregates condition monitoring data, inspection history, and technician inputs into a centralized interface. Supervisors can proactively reassign workloads, order parts, and fine-tune maintenance strategies based on live data.
Case in Point: Indorama Ventures' Digital Maintenance Breakthrough
At its Port Neches facility, Indorama Ventures replaced paper-based maintenance processes with Innovapptive’s Connected Worker Platform to reduce downtime and improve asset reliability. By empowering frontline teams with mobile tools and real-time data, the company achieved projected savings of over $3.3 million annually, including $1.3 million from reduced equipment failures. The shift enabled faster issue detection, streamlined work execution, and a more proactive maintenance culture—demonstrating the real-world value of predictive maintenance at scale.
Beyond Technology: The Organizational Impact
Implementing AI-driven predictive maintenance isn't just about installing sensors or buying software. It requires aligning digital tools with the realities of the frontline workforce. This includes:
- Upskilling technicians to interpret AI alerts and trust data over legacy instincts.
- Creating feedback loops between field operators and AI models to continuously refine predictions.
- Standardizing procedures using digital work instructions that adapt to asset conditions in real time.
Platforms like Innovapptive succeed here because they don’t treat AI as a back-office black box. They bring intelligence to the frontline—where downtime is either avoided or allowed to happen.
Conclusion
AI in predictive maintenance is no longer a futuristic concept—it’s a present-day necessity. Organizations that leverage AI to anticipate equipment failures, automate workflows, and empower their frontline teams will see dramatic reductions in downtime and operational disruptions.
By integrating real-time monitoring, AI root cause analysis, mobile maintenance workflows, and prescriptive insights, platforms like Innovapptive help companies move from a reactive firefighting culture to a proactive, data-driven maintenance strategy.
Ready to reduce downtime and unlock millions in operational savings? Schedule a personalized demo to see how Innovapptive’s AI-powered Connected Worker Platform can transform your maintenance operations.
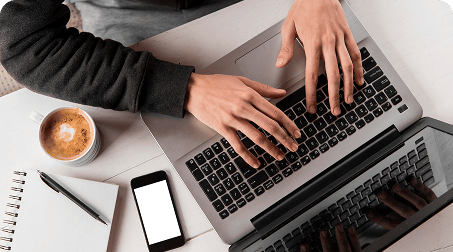
See It In Action
Schedule a personalized demo to see how our solutions can help your business thrive.
- 22-04-2025
The $3.6B Unlock: Solving the Chemical Industry’s Labor Crisis and EBITDA Pressure in One Move
“Constraints don’t slow innovation—they force it.”
- 21-04-2025
Chemical Industry's Margin Erosion: A Wake-Up Call for Operational Efficiency Amid China's Oversupply
The global chemical industry is facing mounting challenges, with revenue declines and shrinking...
- 21-04-2025
Why Wrench Time Isn’t Improving: The Hidden Costs of Traditional Maintenance Scheduling
For years, industrial leaders have relied on a common but flawed assumption: "Better planning and...